numpy 데이터를 DB 삽입 하려고하다보니
형식이 맞지않아 삽입을 할 수 없는 상황이 생겼다.
그래서 Python의 flaot 형태로 변경해서 삽입하려고 시도했고
numpy 에서 tolist() 함수를 통해 Python float 형태로 변경하면 FP형식이 유지되지않는다.
**numpy FP16을 Python으로 변환해도 FP32로 바뀌어서 변환된다.
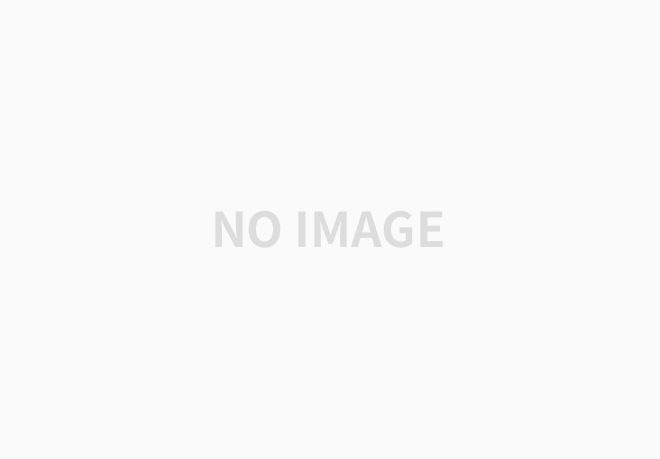
그래서 numpy 형식을 python float32 형태로 변경하고 그것을 round 함수를 통해 4째자리까지만 잘라내려고한다.(FP16)
총 256차원의 7,000,000만건의 벡터(Vector) 데이터를 전처리해야했고 생각없이 코드를 작성했더니
많은 시간(10분이상)이 소요되었고 중간중간 병목현상이 발생하였다.
이때 개념으로만 알고있던 멀티 프로세싱이 떠올랐고 그것을 실제로 전처리에 적용해보았다.
단일 프로세싱
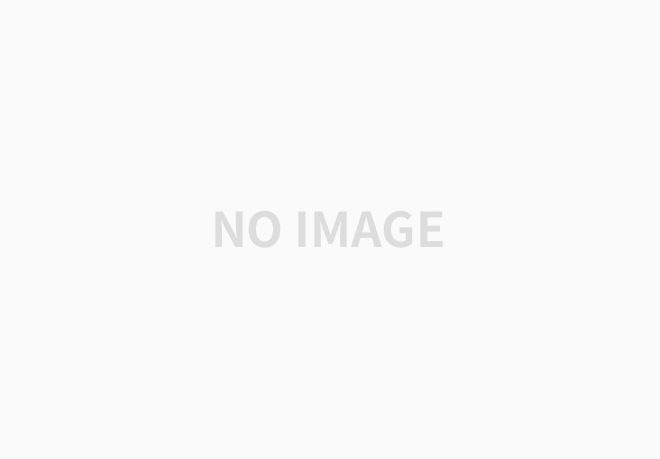
단일 프로세스를 사용할 경우 저렇게 8번 cpu와 약간의 10번 cpu가 사용된다.
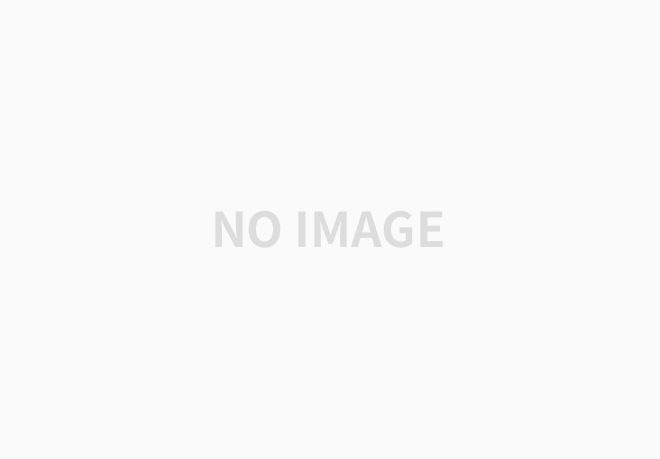
tqdm으로 모니터링했을때 예상 시간은 5분 27초 이지만 중간중간 병목현상이 발생하여 실제로는 10분이상 소요되었다.
멀티프로세싱
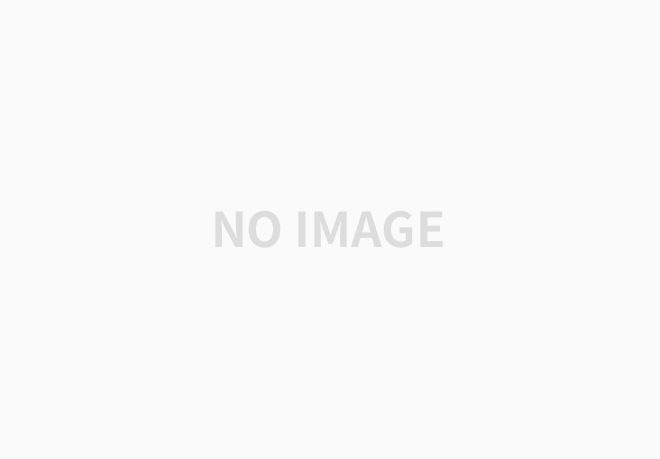
멀티프로세싱을 사용해서 24개의 cpu 중에 16개를 사용하여 함수를 실행시켰다.
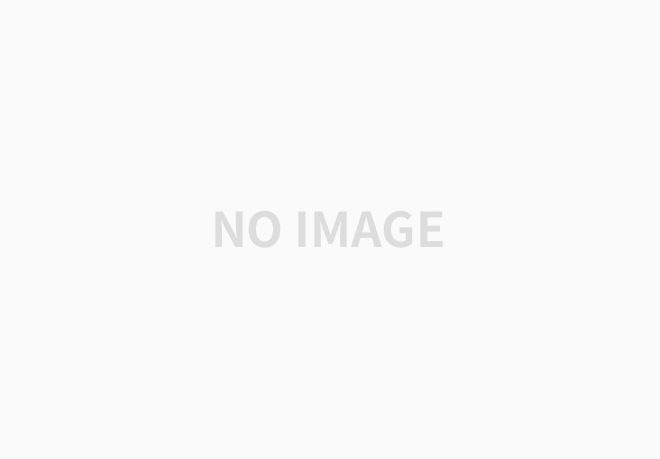
7,000,000개의 데이터를 16등분(n_cpu=16)하여 435441로 나눴고 각각의 CPU가 일을처리하는 모습을 볼 수 있다.
10분에서 -> 1분으로 시간이 1/10으로 줄어들었다.
좋은 컴퓨터 가지고 왜 이걸 이제 알았을까 후회되었지만 이제라도 알았으니 알차게 CPU를 사용해야겠다.
코드 구현
import numpy as np
from concurrent.futures import ProcessPoolExecutor
import multiprocessing
import itertools
import time
from tqdm import tqdm
n_cpu = int(multiprocessing.cpu_count() * 0.7) # 내 컴퓨터 CPU 코어 수 * 사용량(0.7)
# ex int(24 * 0.7) == 16
numpy 데이터 로드
embedding_npy = np.load('./lassl_embedding_float16.npy')
변환 함수
def numpy_to_plist(start, end):
tmp = []
for i in tqdm(embedding_npy[start:end]):
temp = []
i = i.tolist()
for j in i:
temp.append(round(j, 4))
tmp.append(temp)
return tmp
멀티 프로레싱 함수
def func_multi():
global embedding_npy
full_len = len(embedding_npy) # 데이터 수
process_index = int(full_len / n_cpu) # 분할 범위
rng_list = [(i + 1) * process_index for i in range(n_cpu)] # 분할 범위 리스트 생성
if rng_list[0] != 0: # 맨앞에 0 추가 ( 0부터 시작해야함 )
rng_list.insert(0, 0)
if rng_list[-1] < full_len: # 맨 뒤는 총 데이터수 추가
rng_list.append(full_len)
with ProcessPoolExecutor(max_workers = n_cpu) as executor:
flaot16_list = list(executor.map(numpy_to_plist, rng_list[0:-1], rng_list[1:]))
result = list(itertools.chain.from_iterable(flaot16_list)) # 코어별로 나눠진 리스트를 1차원으로 합침
return result
주의!! 함수실행은 main 블록안에서 실행해주여야함.
if __name__ == "__main__":
func_multi()
concurrent.futures.process.BrokenProcessPool: A process in the process pool was terminated abruptly while the future was running or pending. 이 에러는 n_cpu 수 즉 worker 수를 줄이면 해결된다.
+ tqdm 추가 예제
import time
import concurrent.futures
from tqdm import tqdm
def f(x):
time.sleep(0.001) # to visualize the progress
return x**2
def run(f, my_iter):
with concurrent.futures.ThreadPoolExecutor() as executor:
results = list(tqdm(executor.map(f, my_iter), total=len(my_iter)))
return results
my_iter = range(100000)
run(f, my_iter)
https://stackoverflow.com/questions/51601756/use-tqdm-with-concurrent-futures
'Python' 카테고리의 다른 글
[AWS]. S3 Bucket 에서 데이터 다운받기 (with Python) (0) | 2023.02.15 |
---|---|
[Python] .파일 실행시 인자값 전달하기 (argparse) (0) | 2023.01.04 |
[Python]. 현재 파일 디렉터리 절대경로 불러오기 (0) | 2022.09.01 |
[날짜]. datetime으로 무슨 요일인지 구하기 (0) | 2022.08.20 |
[교육자료]. 파이썬으로 행맨(Hangman) 게임 구현하기 (0) | 2022.05.14 |